Every day, telecom operators wield a double-edged sword. They are fortunate to be in a business that thrives on data—the industry collects more customer data on average than just about any other. Yet this data can also be a curse. Customers for the most part know their data is being collected, and are beginning to expect companies to use it to improve experiences, products, services, and the like. In addition, there are a number of substantial IT, business, and privacy issues surrounding the effective use of data.
When it comes to data strategy, how can companies turn the information they have into real actionable insight? What’s hot, what’s hype, and what can make a real impact on business?
Analytics is already a key component of telecom businesses, and it’s poised to grow substantially. Today, the industry invests approximately $1.9 billion in analytics that are primarily descriptive and diagnostic. Firms look at past interactions to make future decisions based on what happened and why. This strategy is evolving, however, to be more predictive and prescriptive. Investment is forecast to reach $9.8 billion by 2020, with the sector connecting more than 11 billion devices, up from 7 billion today, according to research from Huawei and IDATE Consulting and Research. Data savvy operators who implement efficient analytics processes will gain significant ground over their competition during this period of growth by creating superior products, services, and experiences.
Advanced analytics can give forward-thinking operators a huge competitive advantage when it comes to meeting the needs of emerging customer groups, facing unforeseen competition, and innovating with new technologies, like mobile broadband. Examples from around the globe include:
- Engage new segments (youth, expats) with relevant offerings
- Improve customer experience by prioritizing customer needs
- Maximize efforts to retain most valuable customers
- Dedicate resources toward customer acquisition to keep revenue high
- Increase focus on customer mobile data use and patterns
- Build a well thought-out mobile data strategy
Three specific types of advanced analytics will help operators face these challenges: geomarketing, social network analysis, and deep packet inspection.
Geomarketing
Geomarketing analytics enables telco operators to use geographical insights to plan marketing and sales activities and get more granular with their analytics activities. It provides an added dimension when profiling customers to better interact with them.
Typical analytics activity such as segmentation, propensity models, and churn strategies take on a new spin. Regional customer segmentation may result in different segmentation thresholds or even new customer profiles. Regional propensity models can reveal region-specific product needs and behaviors that will initiate new marketing actions, and regional churn models may uncover unique drivers of churn, which can be fine-tuned in more relevant, local customer retention programs and communications.
Peppers & Rogers Group has created a framework specifically for geomarketing within telecoms (see Figure 1). First, you need to understand the overall company strategy and goals—which segments to focus on, what products to prioritize, and how much market share to gain or protect. Measurement is critical in this phase. Identify relevant KPIs to track customer value, needs, and behavior. Design reports and dashboards for senior leaders and other stakeholders that include geomarketing elements. Involve IT in this critical planning stage to pinpoint data to be analyzed from the necessary platforms and create new data efforts if necessary.
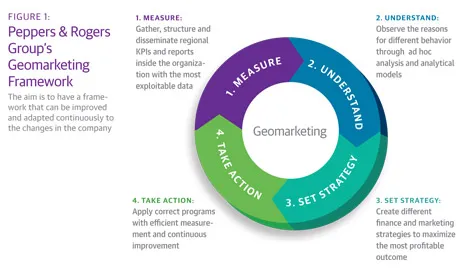
With the foundational elements in place, the next step is to capture insights to understand the effect of geomarketing. This can be done by enriching segmentation programs with location information to understand the distribution of different profiles in different categories, as well as the value and behavior of customers in those areas. There is opportunity to apply propensity models and churn analysis to different locations to test and learn what products, services, and promotions work best, and if location is a potential root cause of churn. The customer experience in different regions can also be assessed to understand unique attributes that influence the customer experience in different geographies.
Then, set the strategy by creating different finance and marketing strategies to maximize the most profitable outcome. It will be possible to prioritize investments based on location value, such as optimizing cell tower maintenance and expansion in high-value areas, or adjusting dealer revenue targets in certain locations. You will have data to back-up operational decisions to change or close low-performing stores. The insight generated will also inform how best to enhance the customer experience in key locations, and marketing efforts can become more granular to manage and grow customer relationships, such as promoting products in potentially valuable locations.
These strategies must then be translated into a diverse set of actions to be implemented across locations. Propose cross-up-sell deals to customers in a specific segment and location with optimized prices. Create retention plans that deter local churn causes in advance. Use the information to improve the customer experience. Know what customers like and do not like in a specific location, and disseminate this information to all channels to provide a consistent experience. Tell customers when an interaction is based on their location. Something as simple as referring to a person’s location in a campaign will likely improve results.
Geomarketing in action: Many mobile operators already analyze customer data to inform one-to-one campaigns for prepaid customers. However, it may take up to six months to understand a customer’s recharging cycles. Knowing the location of the customer at a very granular level may shorten this learning curve because operators can look at similar habits of other customers in that region. Profiling new customers according to their demographic segment and location can let the mobile operator address the customer with campaigns from the first month.
Social network analysis
Understanding relationships among the customer base allows operators to get a better sense of their customers and how best to interact with them. Social network analysis (SNA) is the analysis of relationships among people, groups, and organizations. Understanding what communities they belong to and how active they are, whether they’re an influencer within their networks, etc. gives operators insight for more relevant and better targeting, enhanced customer differentiation, and a more complete customer value analysis.
Social network analysis is not social media analysis. It leverages the valuable data being collected by telecoms to gain a true customer value picture. It starts with a customer’s ARPU (average revenue per user). Then, traditional calling circle analysis can determine the connections per user. Add to it elementary metrics like the number of connections and density of out-of-network calls to derive and visualize a customer’s actual social network.
A unified ‘intensity’ measure represents the strength of ties between metrics like call frequency and duration. The user’s connections are ranked based on this intensity metric. Each user is profiled based on his immediate contacts, and social boundaries are drawn. A person’s social network then comes into focus. And the value of his or her network is determined by the activity of connections within the network and their ARPU (see Figure 2).
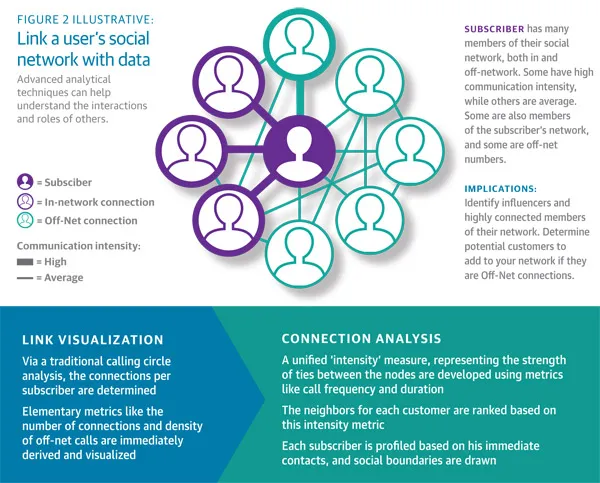
This analysis can be used to identify high priority customers for acquisition based on network value and contribution. Attrition can also be predicted by gauging the pressure on communities when one of their members churns. SNA works on the premise that a churner exerts pressure on other community members to churn, as well.
Finally, SNA can improve marketing success through the definition of target groups based on the size of their networks and their roles within it. Influencers are people who are more (or more strongly) connected, trailed by neutrals and followers. Not only will influencers’ impact on the network be larger than for people with an average amount of connections, they are also an ideal target for a marketing campaign.
Social network analysis in action: Peppers & Rogers Group worked with an operator to assess customer churn pressure and churn influencer scores using the SNA model. The team identified churners with high influence scores, and analyzed the behavior of their social network. The analysis revealed that the probability of finding another churner(s) in a churn influencer’s social network is six times more likely than finding another churner(s) in the social network of an ordinary churner. With this insight, we piloted a few campaigns to its influencer segment to head off potential churn. More than 50 percent of the influencer group opted in to the campaigns, compared to less than 25-percent opt-in rate from mass campaigns.
Deep packet inspection analysis
Mobile broadband is becoming a dominant communication platform worldwide. Operators, which provide the infrastructure for these systems, have the opportunity to get a deep understanding of what and why customers are using mobile broadband data.
Deep Packet Inspection (DPI) is a next-generation technology capable of inspecting every byte of every data packet transmitted over an operator’s network. DPI devices can examine packet payloads to identify the program or service being used by customers. Operators may use the available data in a number of ways, including for customer engagement and customer experience improvements.
On the engagement side, telcos can introduce innovative content-based plans to meet the needs of individual customers, study overall network usage to analyze aggregate behavior, and identify behavior patterns by application type and time of day/week to engage customers with the right offer at the right time. By analyzing aggregated data, operators can also determine the exact applications used on their network (see Figure 3). In addition, operators can ensure service quality by instigating traffic control and bandwidth allocations, prioritizing popular applications such as YouTube, Netflix, or Skype, over other less time sensitive data. And from a revenue generating perspective, operators can create plans with different service levels enforced through DPI tools.
On a more granular level, DPI data enables operators to segment customers by their online behavior. For example, “VoIP Chatters” browse within normal levels, but use above average Skype, WhatsApp, or other communication apps, while “Energetic Youths” mainly use their data plans for gaming and video streaming. This insight can lead to new product and service bundles based on segment needs, moving away from mass market plans.
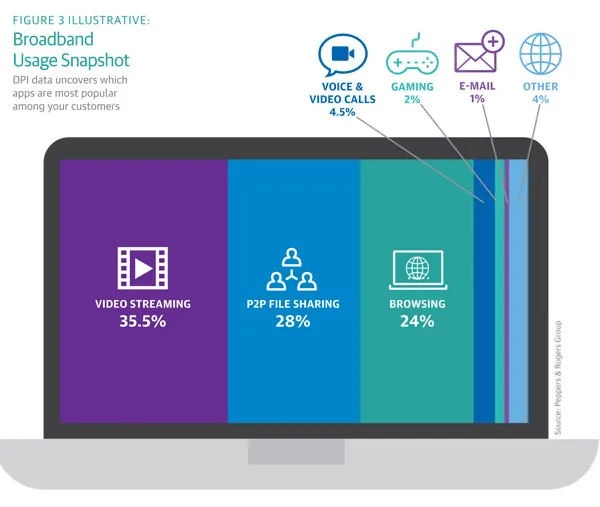
DPI analysis in action: One leading operator offers an unlimited social data package, allowing subscribers to access only Facebook, Blackberry Messenger, Twitter, and WhatsApp through their mobile devices. Similar packages can be developed that include email, video streaming, or P2P usage.
Challenges and roadblocks
Building an effective advanced analytics capability within an organization requires long-term vision, planning, and persistence. It is a gradual process from designing an exhaustive data management environment to implementing analytics solutions for decision making.
The most basic requirement for analytics is data, which requires setting up a robust IT infrastructure. This requires millions of dollars of investment in the form of secure IT systems, analytics software, and skilled human resources. Since analytics is a fairly new industry, companies are wary of making a big investment due to lack of analytics know-how and conviction. This is the first challenge that executives must address on their path to analytics maturity.
With analytics bringing in huge monetary value to businesses, companies around the globe are taking notice and analytics adoption is growing at a tremendous pace. However, very few of these companies are successfully able to maximize its potential due to a dearth of analytics talent, an out-of-sync marketing ecosystem, or both. This is the second hurdle to be overcome, and requires a well-defined organizational structure and efficient company processes. With either of these elements missing, any analytics initiative is doomed to fail at either the development stage or the implementation stage.
In their quest for analytics maturity, leaders must be cognizant that the industry is constantly evolving and it is of utmost importance to continuously innovate and persevere.
Conclusion
While each of these individual analytics tactics provide important insights, it is critical to adopt all three to be able to use insights to their maximum value. In this case 1+1+1 will equal 6, as each helps accentuate the impact of the others.