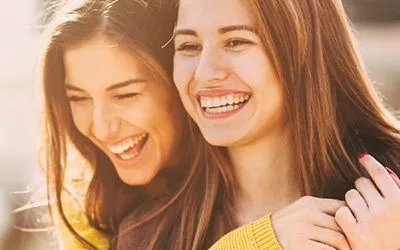
Crystal ball? No. Predictive analytics? Yes
Our client was transitioning from a product-led culture to one designed around service. Our job was to help them better understand the customer journey and the risk factors for customer churn.
Crystal ball? No. Predictive analytics? Yes
Our client was transitioning from a product-led culture to one designed around service. Our job was to help them better understand the customer journey and the risk factors for customer churn.
business program
collection and use
of customer data
enabled personalized
customer interactions
This global technology giant has taken a new perspective on innovation, and this time products are not the focus. The company began a transformation from a product-led culture to one designed around service. To support this customer-centric focus, TTEC was brought in to create a customer retention and renewal program for the company’s new cloud-based subscription model. Long-term relationships were critical to the success of the program; our job was to help the company’s newly formed product intelligence group better understand the customer journey, and proactively identify and address risk factors for customer attrition.
Predictive analytic models are crucial for helping companies understand what customers may do in the future; however in order to accomplish this, there needs to be a clear understanding of the customer’s past and present journey. The client only understood parts of each user’s history, and the data was not collected into one holistic customer view. Once the company could understand what data needed to be measured and where it could be located, the right information could be pulled together into a sophisticated customer segmentation model.
Customers churn due to cost, value, need, or experience. By understanding how these factors differ with each customer segment, the company can now communicate in a more personalized way with customers in order to increase retention. As customers move through their life cycle, the data generated from their interactions is used to gather meaningful insights that refine the predictive models. The customer intelligence strategy becomes continuously self-improving, which improves the effectiveness of each customer’s treatment.
The client’s product intelligence group continues to build the capabilities to anticipate, understand, and predict customer needs, and can now more clearly identify the best action for each account. Although it’s too early to garner any results, the initial launch led to fast growth, and the team is creating a common set of metrics for the company to use on other initiatives, bringing the customer into permanent focus.